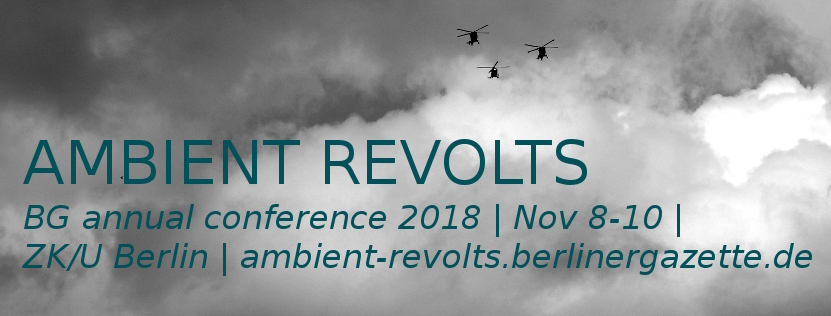
Krystian Woznicki:‘Rethinking political agency in an AI-driven world’ is the topic of the AMBIENT REVOLTS conference in Berlin on 8–10 November. I would therefore like to begin by asking you about the deployment of algorithms at state borders. You have noted that ‘in order to learn, to change daily and evolve [they] require precisely the circulations and mobilities that pass through’. This observation is part of your larger argument about how governmentality is less concerned with prohibiting movement than with facilitating it in productive ways. The role of self-learning algorithms would seem to be very significant in this context, since – like capitalism – they also hinge upon movement. What does it mean for you to think about the relationship between self-learning algorithms and capitalism when it comes to their thirst for traffic?
Louise Amoore: Yes, I agree that the role of ‘self learning’ or semi-supervised algorithms is of the utmost relevance in understanding how movement and circulation matters. First, perhaps it is worth reflecting on what one means by ‘self learning’ in the context of algorithms. As algorithms such as deep neural nets and random forests become deployed in border controls, in one sense they do self-learn because they are exposed to a corpus of data (for example on past travel) from which they generate clusters of shared attributes. When people say that these algorithms ‘detect patterns’, this is what they mean really – that the algorithms group the data according to the presence or absence of particular features in the data. Where we do need to be careful with the idea of ‘self learning’, though, is that this is in no sense fully autonomous. The learning involves many other interactions, for example with the humans who select or label the training data from which the algorithms learn, with others who move the threshold of the sensitivity of the algorithm (recalibrating false positives and false negatives at the border), and indeed interactions with other algorithms such as biometric models.
I do think that the circulations and mobilities passing through are extraordinarily important conditions of possibility for algorithms at the border. Put simply, there are no fixed criteria or categories for what ‘normal’ or ‘anomalous’ might look like, no fixed notions of what kinds of movement are to be prohibited. Instead, there is a mobile setting of thresholds of norm and anomaly which is always conducted in relation to the input data to the algorithm. For example, deep learning algorithms are increasingly being used to detect immigration risks and to detain someone in advance of reaching the border (e.g. in visa applications). The decision about that person is made not primarily in terms of their own data but more significantly in relation to an algorithm that has learned its risk thresholds by exposure to the attributes of vast numbers of unknown others. There are profound consequences for ethico-politics – the algorithm that will detain some future person has learned to recognise on the basis of the attributes of others. The thirst for traffic, as you put it, is a thirst for the data that fuels the generation of algorithmic models for border control.
The relationship between machine learning and capitalism is interesting, of course. In my book The Politics of Possibility, I described how a set of algorithms designed for commercial consumer methods ultimately became a resource in the so-called war on terror. In effect, the commercial target of an ‘unknown consumer’ (someone not yet encountered but who may have a propensity to shop in a particular way, for example) became allied to ideas of the ‘unknown terrorist’ (someone not yet encountered who may have a propensity to be a threat). To the algorithm it does not matter whether the target is for capital or for the state, it is indifferent in this sense. I do think that this relationship between machine learning and capital continues to shift and change. For example, the Cambridge Analytica algorithms were used in commercial and political spheres and in both cases the target output for the algorithm was a propensity to be influenced in a specific way by targeted media. There has been public outcry at the effects of such algorithms on the democratic process – particularly in the Brexit referendum and the election of Trump – but similar algorithms are being used every day to police cities, to stop or to detain people at multiple borders, from railways stations to shopping malls.
It seems what we are dealing with here is the systematization of the possible: the target is something unknown that, by way of systematization, becomes a circumscribed and definite possibility – or rather an array of definite possibilities. The deployment of algorithms is thus ‘mobile’ and ‘flexible’ but, because it is oriented towards definite possibilities, not as much as it may seem; in fact there is only a definite and therefore limited spectrum of possibilities that algorithmic modelling can address. Does this situation change in the face of self-learning algorithms, given their inductive capacities to create semi-autonomous associations in an ever-growing range of possibilities?
The orientation of algorithms towards definite possibilities is important, yes. In one sense I agree that there is only a limited range of possibilities. Let us make this a little more concrete with an example. One group of algorithm designers whom I observed for my research explained to me how they modify their model according to the output. Their algorithms are used in a wide range of applications, from surgical robotics to gait recognition and detecting online gambling addiction. When they set a target output, this is indeed a kind of limited spectrum of possibilities – the target has to be a numeric output between 0 and 1. However, the distance between the actual output signals of their algorithms and the target output represents what I call a space of play. Indeed, the algorithm designers described ‘playing with’ or ‘tuning’ the algorithm so that the output converges on the target. Here I think that deep machine learning is not circumscribed at all by a limited spectrum of possibility. A minute change in the weights inside one layer of the neural net can shift the output of the algorithm dramatically. In a convolutional neural net for image detection or face recognition, for example, this can represent millions of possible parameters, far in excess of what could be meaningfully understood by a human. This is why I am sceptical of claims about ‘opening the black box’ of the algorithm in order to have some kind of accountability. I would say instead that there is no transparency or accountability in the algorithm’s space of play, and so we must begin instead from notions of opacity and partiality.
If the systematization of the possible is somehow reconfigured by self-learning algorithms, are the capacities of indefinite potential then also reconfigured, for example when it comes to evading this very systematization? In other words: is a new, ‘intelligent’ systematization of indefinite potential arising in the context of AI?
You have really identified a crucial issue here. In the final chapter of Politics of Possibility, I proposed that potentiality continues to overflow and exceed the capacity for the calculation of possibles. However, I am worried that this evasive potentiality may also be under threat, and I do address that in my new book Cloud Ethics. With contemporary deep machine learning, there is a move to incorporate the incalculable and to generate potentials that need never be fully exhausted. Gilles Deleuze once wrote that ‘the problem gets the solution it deserves’, implying that the particular arrangement of a problem will systematize a solution. To my reading, today’s algorithms are reversing this, so that the solution gets the problem it deserves – in the sense that the potential pathways of the neural net are infinitely malleable in relation to a solution. Let us not forget that by ‘solution’ we mean an algorithm that may decide juridical processes, policing, security, employment and so on.
An associationism that can never be known, a life of associating with other things and people that is not amenable to and not incorporable by calculation – is this now changing through AI? Is the potential of associating becoming amenable to calculation as AI thrives on an associationism that can never be known completely? In other words: does AI entail a shift in the ontology of association?
A life of associating with other things and people is where I think some of the greatest harms of AI reside. That is to say, for me there is profound violence in the way algorithms redefine how we might live together and decide, uncertainly, in the context of unknown futures. To gather together, to make political claims in the world, to associate with others in the absence of secure recognition – all of this is threatened by AI. And, of course, it has profound consequences. Following the murder of Freddie Gray by Baltimore police in 2015, for example, it was machine learning algorithms that ‘detected hints of unrest’ among the African American population and preemptively targeted associative life. High school students were prevented from boarding buses to join the protest, people were arrested for their social media content, and groups were apprehended on the basis of image recognition. The potential of associating becoming amenable to calculation is something that is real and happening in the world. If there is a shift in the ontology of association with AI, then this is a shift that replaces the conventional ‘association rule’ of old data mining with something like an association of attributes. Attributes do not map onto individuals but onto small fragments of a person’s data, in association with small fragments of another’s, but calibrated against the feature vectors of the algorithm. What this ontology meant for the protestors of Baltimore is that they could not gather together in a public space because their attributes had already gathered in a risk model that can action the incalculable.
You have argued in your work that we are facing new, underacknowledged forms of algorithmic discrimination and prejudice. You note that post-9/11, risk-oriented forms of algorithmic modelling are ‘prejudicial or discriminatory’ but that they ‘write their lines in a novel form that never quite lets go of other future possibilities’. Could you explain how these new forms differ from older forms of technology-based discrimination? What is new about the new?
In many ways, new forms of algorithmic modelling amplify the racialized targeting of black and brown bodies that has for so long circumscribed access to the public sphere and to politics. As Safiya Umoja Noble has documented vividly in her book Algorithms of Oppression, algorithms reinforce racism in distinct ways. One of my concerns, however, is that there has been a growing call for more accountable and ethical algorithms, as though the discrimination and bias could be corrected out or extracted. In fact, machine learning algorithms need assumptions and bias in order to function. They cannot simply have their discriminatory practices modified by, for example, adjusting the training data or the source code.
For me, the significant difference between what you call ‘older forms’ and novel deep learning is the particular relationship between individual and population, and how this is used to govern life. Consider, for example, the racist profiles of Francis Galton’s nineteenth-century composite portraits or Adolphe Quetelet’s statistics of the average man. What were ‘variables’ in these social models would in contemporary terms be closer to computational attributes or features. Consider, for example, the young man who is detained in a police station because the risk algorithm outputs a high score for propensity to abscond. This does not take place because he shares the statistical probability or fixed profile of threat. Rather, it is because the feature vectors of his data have a proximity to features derived from the millions of parameters of the data of unknown others. Yes, the algorithm acts in a way that is racist and prejudicial, but it is in a form that involves new ethico-political relations to ourselves and to others. Very frequently I have heard a desk analyst or police operator say that ‘well, I can just move the threshold if I don’t find a useful output’. The moving of a threshold in the computation is the moving of a relation of societal norms to societal anomalies. Yes, we have seen discrimination and prejudice from the Hollerith machine to the bell curve, but it is crucial that we understand what it means to define a feature or an attribute, to move a threshold or adjust a weight.
Do self-learning algorithms introduce another quality in this context? Or are they merely more efficient when it comes to discriminating indifferently between subjects?
Efficiency is an interesting way to put it. This is what is often claimed for algorithmic systems at the border or in the criminal justice system – to have a more accurate and efficient process of targeting what matters. But, in fact there are all kinds of inefficiencies too. For example, when the UK police forces have used automated facial recognition algorithms to detect target individuals in crowds, the proliferation of false positives – with the corresponding stopping, searching and identifying of people – has been inefficient and discriminatory. So, then what comes to matter? I think that error is an interesting question here. One could point to all of the many errors and say ‘here is the space for critique and potential alternative futures, here in the excess of the errors’. But, again, at the level of the algorithm, error is distance. What does it mean to say error is distance? Error is merely the spatial gap between the output and a target. And so, even error is productive, even error is incorporable within the generative capacities of machine learning. How does one begin to adjudicate on discrimination with indifference when it is the algorithms that are generating the means to adjudicate in the world, to identify good and bad, to filtrate and condense to an optimized output? Yes, perhaps optimization and not efficiency is the heart of it.
In your work there is a notion of active agency attributed to technology and data. With regard to radio frequency identification (RFID), for instance, you speak of ‘ambient locatability’; and with regard to security tech, you explore how this technology ‘lets the environment talk’. Thinking both ideas in conjunction, the notion of ‘ambient agency’ arises – a notion that becomes more complex still when you embark upon a critique of anthropocentrism, noting that ‘species life has so dominated our thinking, that the bio in biopolitics has a blind spot with regard to the lives of objects’. What does it mean for you to rethink human agency against this background?
The question of what it means to be human is paramount here. It is my view that our relations to ourselves and to others is changing through our interactions with algorithms. One of the most striking moments in my recent research was when an experienced surgeon described how using her surgical robot to excise tumours had changed her sense of the limits of her own agency. She definitively did not distinguish between the surgical instruments of the robot, the algorithms that animate the API of the machine, and her human capacities. These were, for her, thoroughly entangled. I found this to be a compelling insight. I followed the design of machine learning for surgery partly because the same families of algorithms are used in autonomous weapons and autonomous vehicles. In terms of ambient locatability, the neural networks locate the edges of tumours, territories, faces, and so on through the data they have been exposed to in training. Because image recognition and language processing uses deep learning, the environment ‘talks’ in new ways. The RFID and other devices I discussed in that book continue to be significant data inputs, but in cloud-based systems they are often one data-stream among many. To rethink human agency against this backdrop implies an acknowledgement of the composite forms of agency that emerge in our entanglements with algorithms and machines. I think that this should be given greater attention in debates about the ‘human in the loop’ supposed to supply the locus of ethics for composite systems such as autonomous weapons. Who is this human? How are their embodied relations to the world changed through their collaborations with algorithms?
As you argue in The Politics of Possibility, algorithmic modelling that is primarily concerned with re-establishing agency is all about generating new capacities to act and intervene in a world of circulation and movement. You undertake a critique of decision making in this context, since these semi-automated sovereign actions are subsumed by what you refer to as ‘actionable analytics’ – indifferent to error, indifferent to the people that are affected by the actions, indifferent to any consequences and life in general. It seems that your critique of this novel form of sovereign agency is primarily concerned with the philosophy of decision. Or are there also other aspects that are important to you when it comes to rethinking political agency in an AI driven world?
I am sure that you are right that I have been preoccupied with the philosophy of decision, and I admit that I am still thinking about this a great deal. To put this into context: very often the public inquiries that have focused on the potential harms of algorithms have expressed concern at what they call ‘algorithmic decision making’. Put simply, the moral panic seems to be about the notion that a machine and not a human decides. Now, setting aside what I have said about all algorithmic decisions containing the residue of multiple other human and machine decisions, what is special about the human who decides? If a human judge decides on a jail sentence without reference to the recidivism algorithm, or a human oncologist decides on a course of treatment without recourse to the optimal pathways algorithm – is it the ‘humanness’ that we value? What is it about this human decision that matters to us? I think that this is interesting because of course the judge, the border guard, and the police officer are fallible, their decisions could later turn out to have been the wrong course of action. Yet, it is precisely this acknowledgment that the decision is made in the dark, in the fully political realm of undecidability, that leaves open the space for other futures, for other pathways not taken. One of my real concerns about the output of a neural net is that it is described as a ‘decision support’ instrument. It does not confront what is undecidable in the world, but makes a claim to the resolution of political difficulties, and in so doing condenses multiplicities to a single output. To be clear, I do not deny that a machine learning algorithm could also be approached critically as an ethico-political agent. I think that we need to ask these kinds of questions: What were the hidden layer pathways not taken in the making of that output? How have a set of contingent weighted probabilities generated something called an automated decision? Can we make those weights in the algorithm actually carry the full weight of political undecidability? We must rethink political agency in an AI driven world, not least because the neural nets in algorithms like those of Cambridge Analytica are remaking political worlds. One place to start, at least for me, would be to insist upon the non-closures and moments of undecidability that continue to lodge themselves within the algorithm.
Louise Amoore will speak on November 8, 2018 at 7:30 p.m. at the AMBIENT REVOLTS conference. More info here: https://ambient-revolts.berlinergazette.de